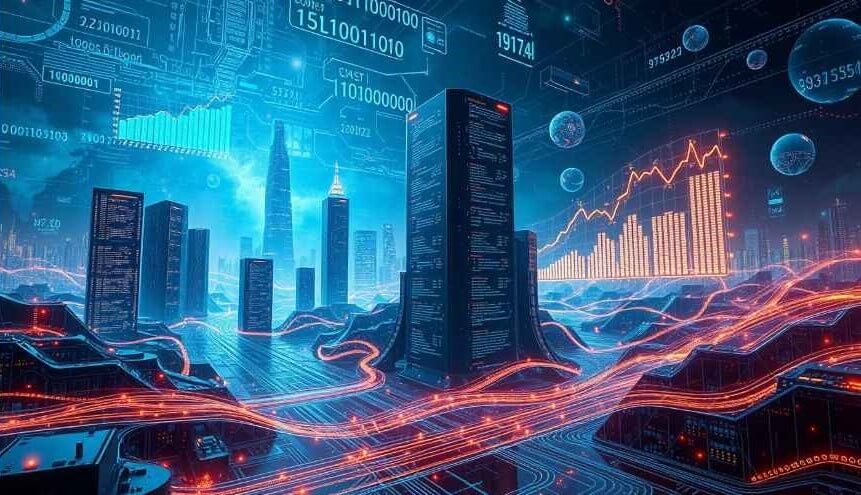
Big Data Technology in Scientific Research
Explore how Big Data Technology is revolutionizing scientific research. Acquire knowledge about the features, significance, and advantages of big data. Discover key big data tools and real-world big data examples in advancing science.
In today’s data-driven era, the role of Big data technology in scientific research has become paramount. These tools and methodologies enable scientists to analyze, store, and interpret massive datasets, uncovering insights that were previously unattainable. This article delves into the characteristics of big data, its importance, and the types of big data used in scientific breakthroughs, while also exploring opportunities and challenges in the field.
1. What is Big data technology?
Big data encompass tools, frameworks, and methodologies designed to handle large and complex datasets that traditional systems cannot manage. From healthcare to environmental studies, these technologies have transformed the research landscape.
Key Characteristics of Big Data:
- Volume: Describes the enormous volume of data produced each second.
- Velocity: The rate of data processing and generation.
- Variety: Different types of data, including structured, unstructured, and semi-structured.
- Veracity: Ensures data accuracy and reliability.
These traits define how big data can be effectively used in scientific endeavors.
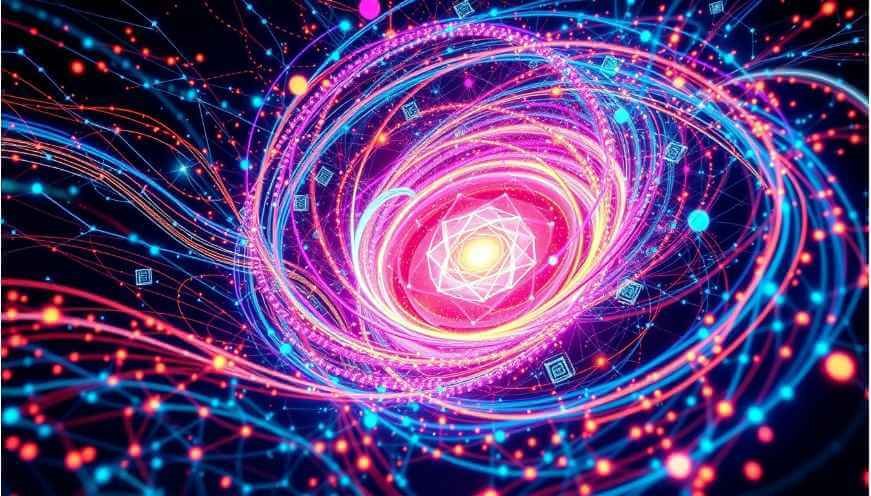
2.The Importance of Big Data in Research
The importance of big data in scientific research cannot be overstated. It enables researchers to explore new possibilities, enhance decision-making, and solve complex problems.
Why Big Data Matters:
- Accelerates Discovery: Processes large datasets quickly to uncover patterns and correlations.
- Enhances Collaboration: Allows researchers from different disciplines to work on the same datasets simultaneously.
- Improves Accuracy: Advanced analytics reduce errors and increase the reliability of research outcomes.
These benefits of big data underscore its significance in addressing modern scientific challenges.
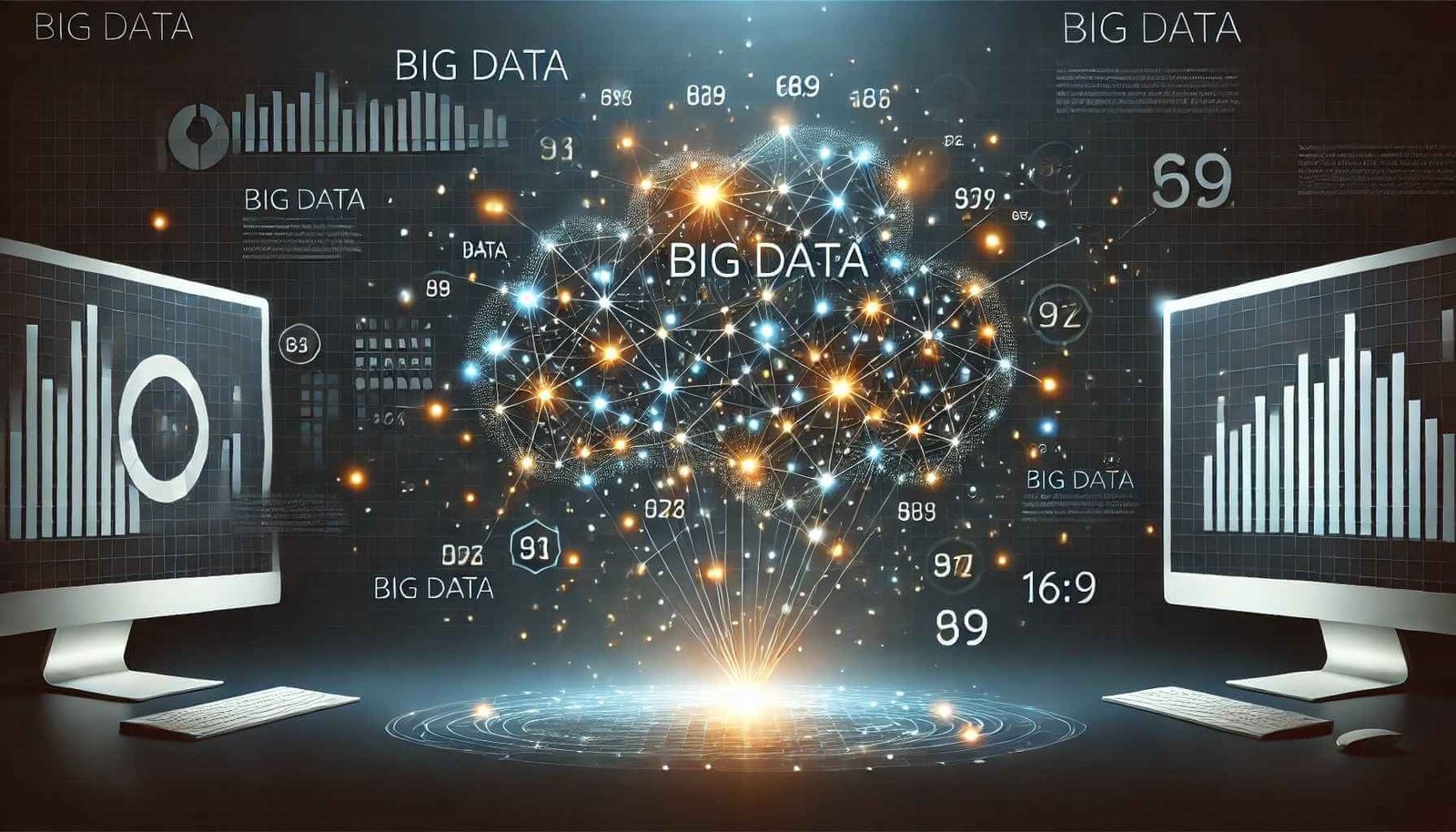
3. Types of Big Data in Science
Understanding the types of big data is essential for its effective application in research. Each type serves different purposes and requires specialized tools.
Common Types of Big Data:
- Structured Data: Organized data like tables and spreadsheets (e.g., genomic sequences).
- Unstructured Data: Includes images, videos, and text, often requiring advanced analytics.
- Semi-Structured Data: Combines elements of both, such as XML files or JSON data.
These categories highlight the diverse nature of data managed by Big data technology.
4. Real-World Big Data Examples in Research
Big data examples showcase how this technology drives innovation and discovery across disciplines.
Key Use Cases:
Healthcare:
- Analyzing patient data to predict disease outbreaks and personalize treatments.
- Big data tools like Hadoop are used for processing electronic health records (EHRs).
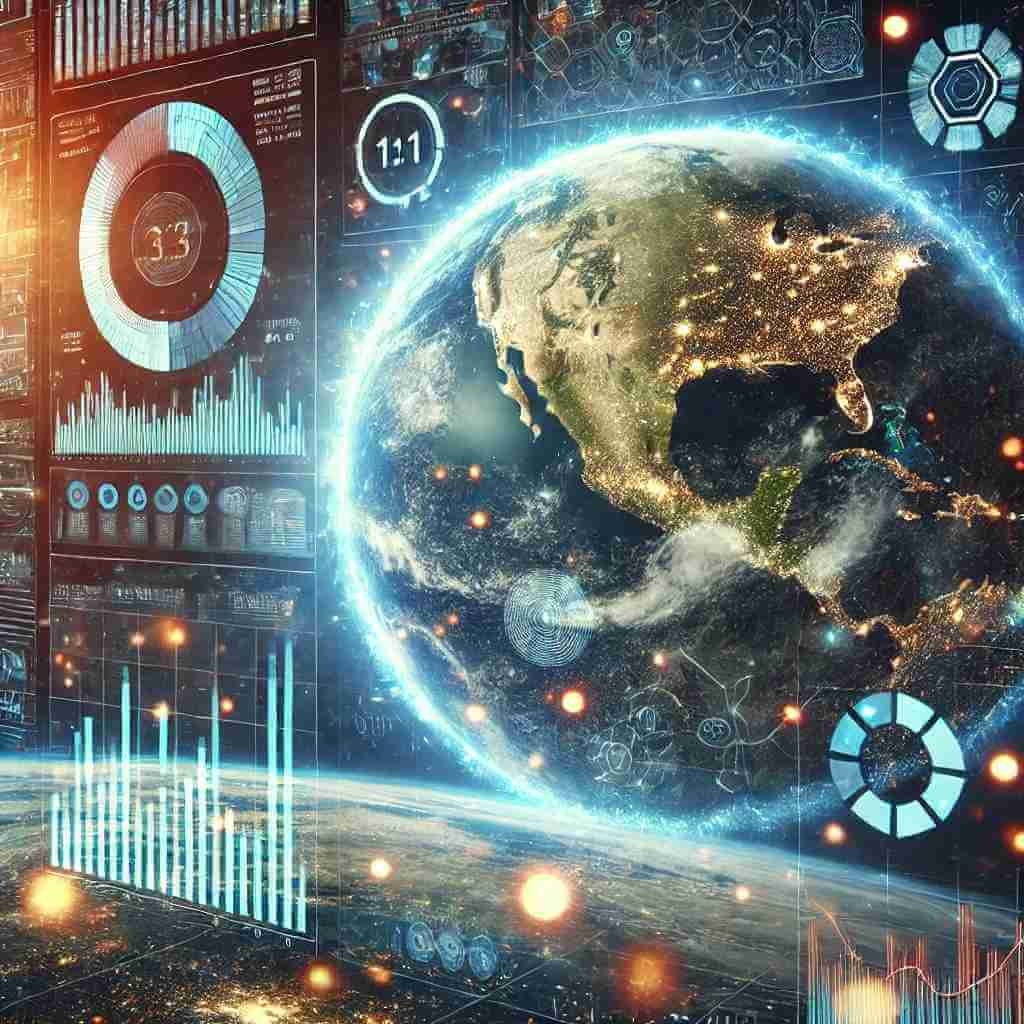
Environmental Studies:
- Monitoring climate change using satellite imagery and IoT sensors.
- predicting and reducing calamities through real-time data analysis.
Physics and Astronomy:
- Processing data from particle accelerators like CERN’s Large Hadron Collider.
- Examining cosmic data to comprehend the beginnings of the universe.
These applications highlight the importance of big data in advancing human knowledge.
5. Benefits of Big Data in Scientific Research
The benefits of big data extend beyond faster processing and deeper insights, creating a transformative impact on how research is conducted.
Advantages:
- Resource Optimization: Allocates computational and human resources more effectively.
- Enhanced Predictive Models: Machine learning models trained on big data improve accuracy in predictions.
- Better Decision-Making: Data-driven insights enable well-informed policy-making and strategy development.
These advantages make Big data technology a cornerstone of modern research.
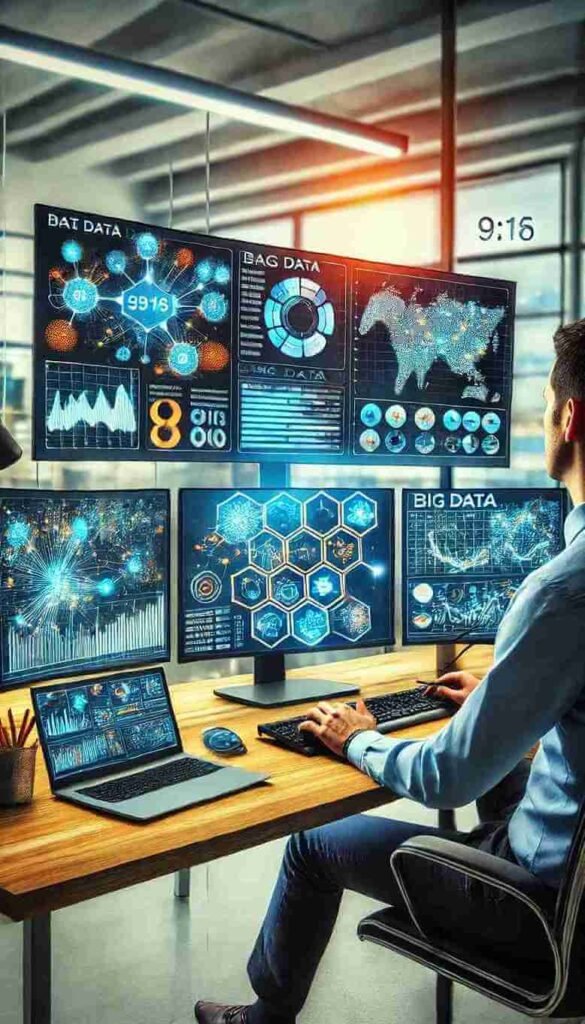
6. Tools and Frameworks for Big Data
To leverage the full potential of big data, researchers use specialized big data tools and platforms.
Popular Tools and Frameworks:
Hadoop: Open-source framework for distributed data processing.
Spark: Well-known in large data analytics for its scalability and quickness.
Tableau: Data visualization tool that simplifies complex datasets.
Python libraries for data analysis and manipulation include Pandas, NumPy, and SciPy.
These tools empower scientists to process, analyze, and visualize data efficiently.
7. Challenges in Implementing Big Data Technology
While the potential of Big data technology is immense, several challenges need to be addressed for effective implementation.
- Common Challenges: Data security is the process of defending private information from hacks and intrusions.
- Scalability Issues: Managing the infrastructure required for processing massive datasets.
- Data quality: Making sure that the information gathered is accurate and consistent.
- Skill Gap: Training researchers to use advanced big data tools effectively.
Overcoming these hurdles is essential to maximize the benefits of big data in scientific research.
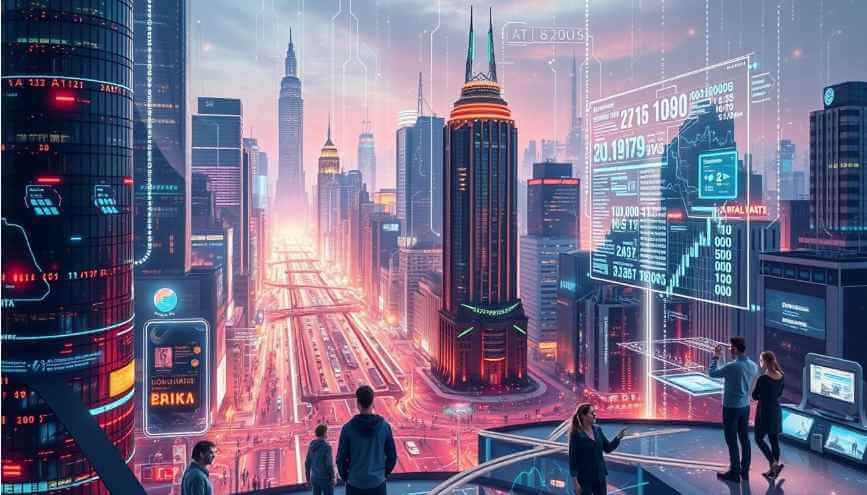
8. The Future of Big Data in Science
The future of Big data in research looks promising, with advancements in AI and machine learning enhancing its capabilities.
Future Trends:
Automated Analytics: AI-driven tools will streamline data analysis and interpretation.
Real-Time Data Processing: Faster data ingestion and insights generation.
Cross-Disciplinary Collaboration: Unified platforms enabling data sharing across scientific fields.
As these trends evolve, the importance of big data will only grow, cementing its role in shaping the future of science.