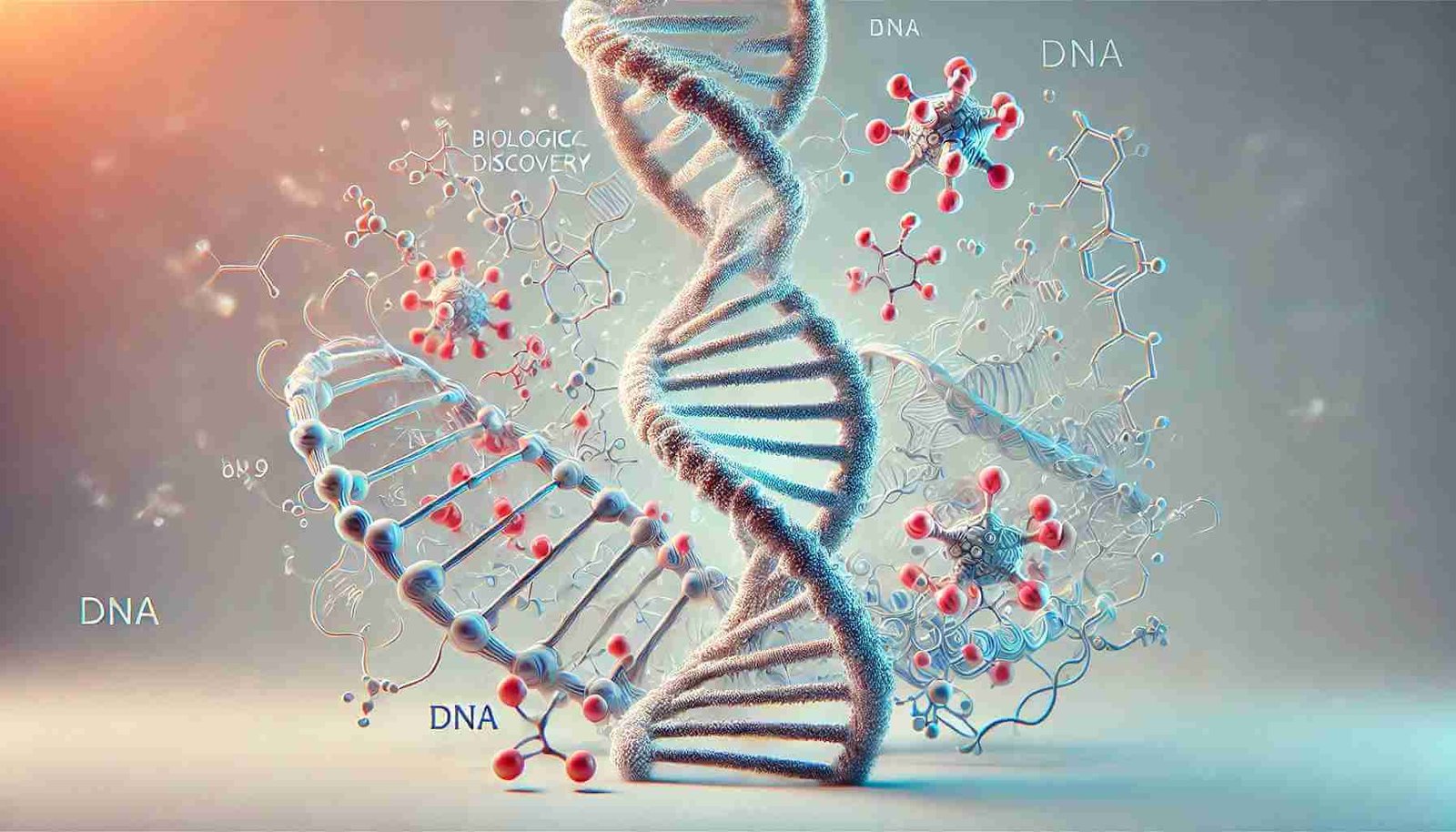
Fig: Generated DNA Image.
AlphaFold3 Open Source Launch: A New Era for AI in Protein Prediction
AlphaFold3 open source release empowers academic researchers with groundbreaking protein-prediction capabilities. Discover its potential impact on drug discovery, competition, and scientific transparency.
After months of anticipation, Google DeepMind’s revolutionary AI-powered protein prediction tool, AlphaFold3, is now open source. This major milestone allows scientists to download AlphaFold3’s code and utilize its advanced capabilities for academic, non-commercial research, sparking excitement and opening new doors for scientific exploration.
The Importance of AlphaFold3 Open Source Launch
The release of AlphaFold3’s code is significant as it marks the removal of restrictions that had previously limited its usage to a web server. While earlier iterations like AlphaFold2 allowed for broad usage and reproducibility by openly sharing code and parameters, AlphaFold3 was initially accessible only through a web platform, restricting certain prediction capabilities—particularly interactions involving potential drug compounds.
DeepMind’s decision to release AlphaFold3 for public use comes on the heels of criticisms regarding the scientific reproducibility of withholding the code. Academic researchers can now freely run AlphaFold3’s model for protein structure prediction, making strides toward a more open scientific community. However, access to training weights—parameters honed through extensive training on protein data—is limited to scientists affiliated with academic institutions, ensuring continued control over commercial use.
A Tool Primed for Drug Discovery
Among AlphaFold3’s most transformative features is its ability to model protein interactions with various molecules, including potential drugs. This capability has vast implications for pharmaceutical research, where predicting protein behavior and binding interactions is essential. Initially, DeepMind limited AlphaFold3’s use by making it available only on a restricted server, which prevented scientists from leveraging the tool’s full capabilities. Now, with the open-source release, AlphaFold3 can be used for in-depth exploration of molecular interactions, laying the groundwork for new treatment possibilities.
The model’s expanded protein-structure modeling functions open up new avenues in understanding molecular dynamics, protein binding, and interactions with candidate drugs. By accurately predicting protein-drug interactions, AlphaFold3 is poised to help researchers identify promising therapeutic pathways and accelerate drug discovery, potentially reducing the time and cost associated with pharmaceutical innovation. Academic scientists now have the opportunity to push the boundaries of drug research by applying AlphaFold3 in ways that could lead to breakthrough therapies.
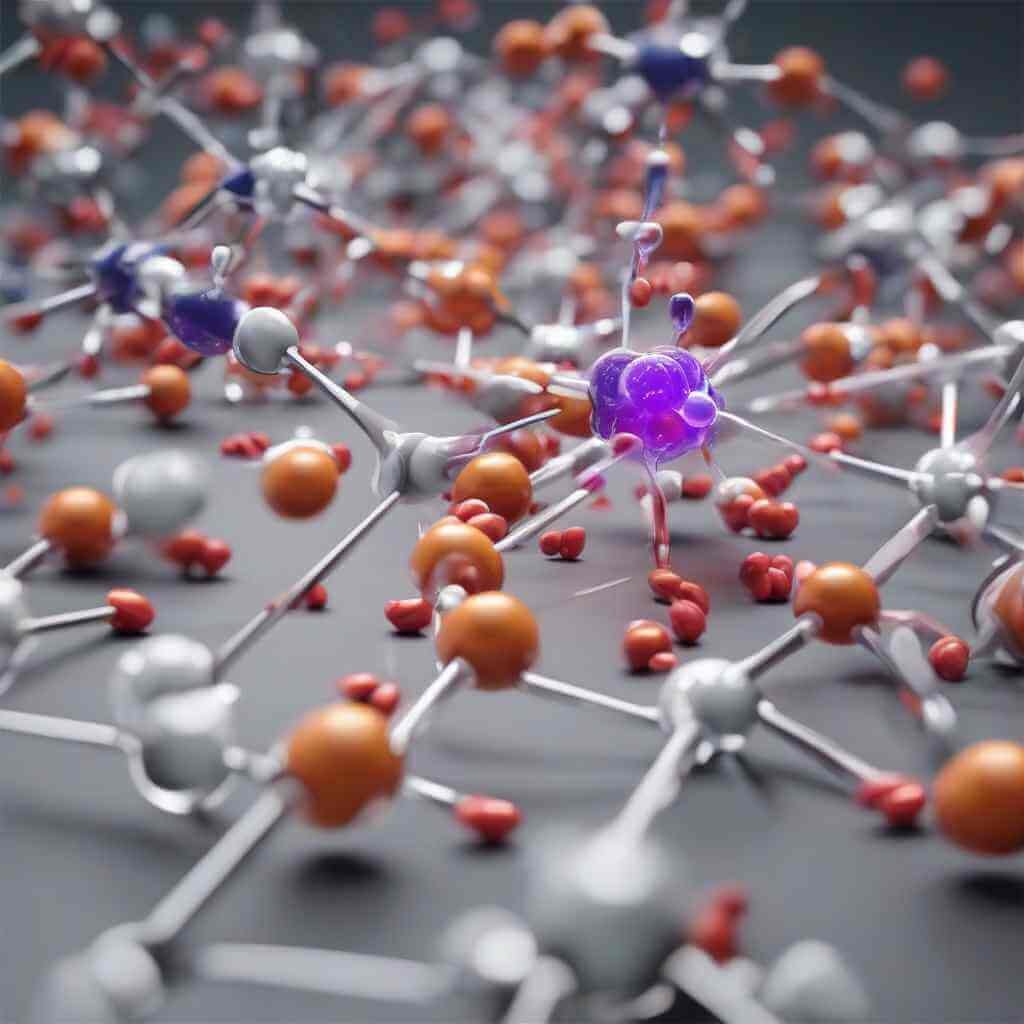
Global Competition from AlphaFold3-Inspired Models
AlphaFold3’s open-source release has spurred a wave of competitive development among other organizations. Major technology firms and startups alike are creating alternative models inspired by AlphaFold3. Chinese tech companies such as Baidu and ByteDance, along with the San Francisco-based startup Chai Discovery, have each developed versions of AlphaFold3 that aim to address different aspects of protein prediction. Chai Discovery, for example, offers a model known as Chai-1, accessible through a web server specifically geared toward drug discovery applications, while Ligo Biosciences has created a model based on AlphaFold3 with fewer restrictions on usage.
However, these models come with their own limitations. Some are still confined to modeling basic protein structures and lack the capability to predict complex interactions with molecules other than proteins. Anticipated models like OpenFold3, set for release by the end of the year, aim to bridge these gaps, enabling drug companies to customize models with proprietary datasets. By allowing modifications, models such as OpenFold3 could further enhance the accuracy and applicability of protein prediction tools, contributing to tailored solutions for various research needs.
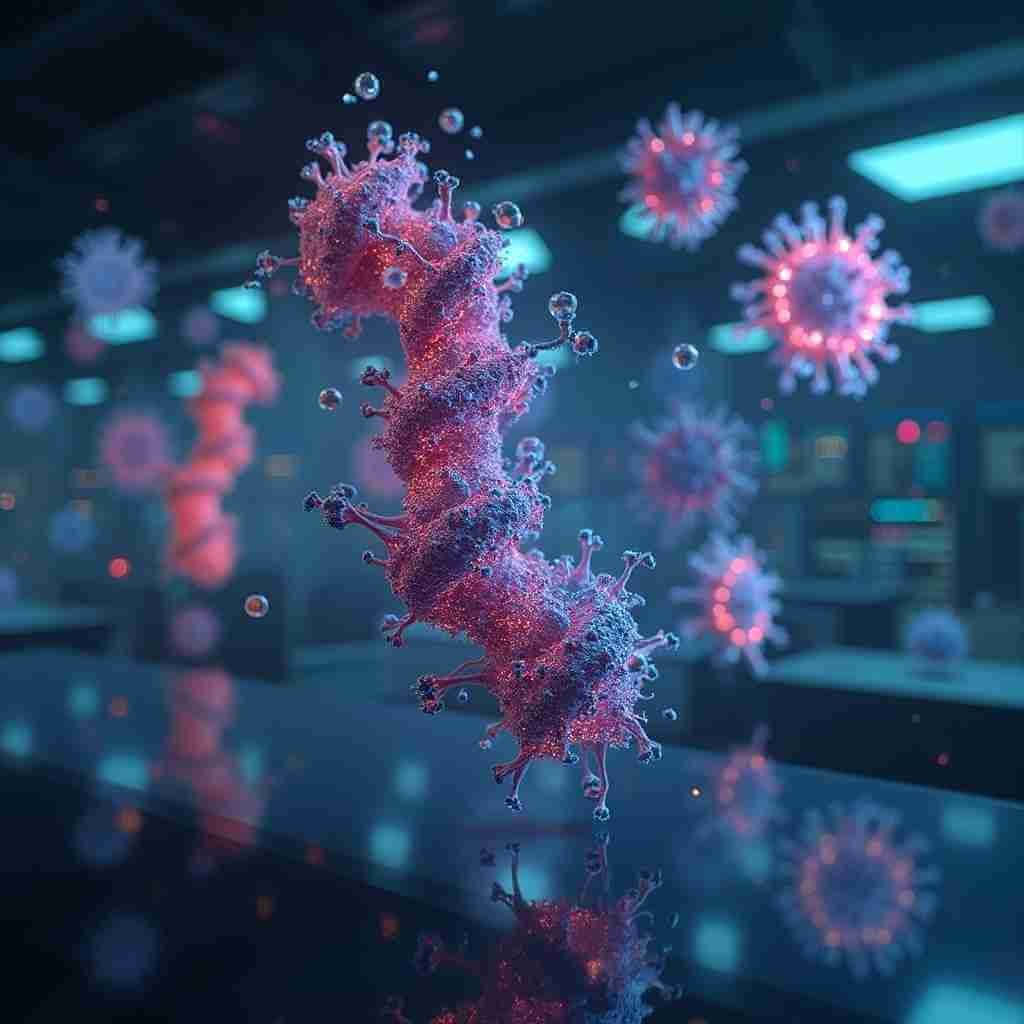
Fig: Concept Art of AlphaFold3
The Importance of Open-Source Innovation in Biological AI
The release of AlphaFold3 highlights a growing trend in the field of biological AI toward open-source models and reproducibility. Open-source AI tools have empowered researchers to refine and build upon foundational models, accelerating innovation in fields like molecular biology. When AlphaFold2 was released openly, it spurred numerous applications within the research community, including novel protein design projects. Some researchers successfully used AlphaFold2 to create proteins capable of targeting cancer cells, while others leveraged it to identify proteins essential for reproductive biology.
Anthony Gitter, a computational biologist at the University of Wisconsin-Madison, emphasizes the role of open-source tools in advancing scientific knowledge. By making models like AlphaFold3 publicly accessible, researchers can inspect, test, and validate the algorithms, ensuring accuracy and reproducibility across studies. Gitter advocates for standardized open-access norms in biological research, encouraging both academic and corporate entities to contribute to a transparent scientific ecosystem.
The Future of AlphaFold3 and Beyond
Pushmeet Kohli, DeepMind’s head of AI for science, notes that the rapid emergence of AlphaFold3-inspired models reflects the model’s potential and reproducibility. He sees the open-source release as a powerful catalyst for future scientific breakthroughs. AlphaFold3 team leader John Jumper also shares this vision, expressing enthusiasm for the innovative and sometimes unexpected ways researchers might apply AlphaFold3 to study protein dynamics and molecular interactions.
Jumper envisions AlphaFold3’s open access leading to novel applications in areas like genetic research, enzymatic engineering, and biochemistry. He is particularly optimistic about scientists using the model creatively to reveal new insights into protein functions, structural adaptations, and molecular behaviors. This broad, collaborative approach could lead to discoveries that extend AlphaFold3’s impact across various biological disciplines.
AlphaFold3’s open-source release represents a significant step toward democratizing protein prediction tools. By making AlphaFold3 accessible to the scientific community, DeepMind is fostering a culture of collaboration, openness, and scientific transparency. Academic researchers now have the ability to explore protein structures with a high degree of accuracy, driving advancements in fields such as drug discovery and molecular biology.
As other companies develop and refine AlphaFold3-inspired models, the potential for innovation in biological AI will only increase, expanding the toolkit available to researchers and accelerating progress in understanding complex biological systems. AlphaFold3’s journey underscores the importance of openness in AI-driven research, and as more breakthroughs emerge, the impact of these open-source tools on scientific discovery is likely to grow, fueling advances in medicine, biology, and beyond.